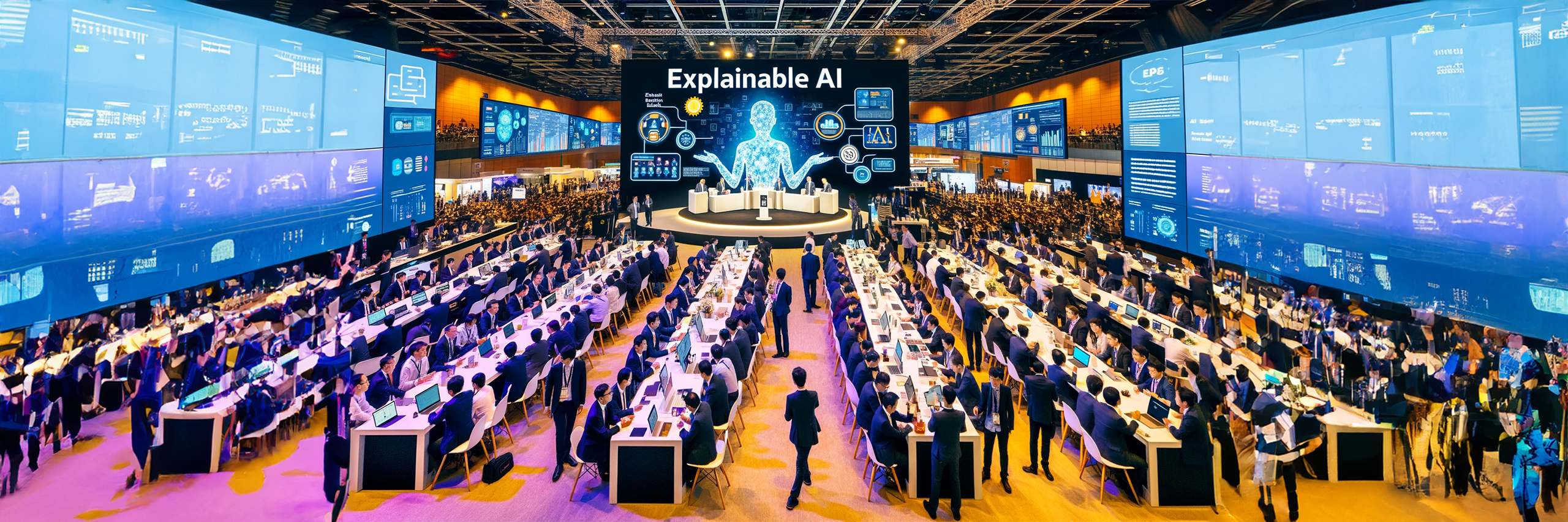
October 2019
Explainable AI is all the rage at legal technology conferences currently. It is considered essential to algorithms that are used in law. Here is why I think that popular view is wrong – and why I generally dislike prediction algorithms anyway.
Machine learning is not Statistics.
There is a popular joke circulating at the moment
“When you’re fundraising, it’s AI.
When you’re hiring, it’s ML.
When you’re implementing, it’s logistic regression.”
Now, behind every joke there is at least an ounce of truth. But the punchline of this joke is only funny if one understands that there is quite a serious difference between deep learning and a simple statistical analysis. The mathematics that we are more commonly familiar with, statistics regressions, a dependent variable, and correlation between different factors, are all what we might typically think of as explainable. Still, they are done entirely differently to machine learning. For example, we might calculate a relationship between inflation and unemployment, or education and life-time earnings, and therefore draw conclusions based on those relationships. Under good scientific analysis, these regressions will be repeatable, with the assumptions made in calculating them will be explainable, and therefore we have the possibility of high-level transparency if we make decisions based on these regressions.
Machine learning, on the other hand, is often described as more art than science. Instead of picking factors by reason of their logical relationship (i.e., not data mining), machine learning will often use a huge number of variables that may not necessary have a direct cause or relationship and will adjust the model-based purely on “what works.” The process of machine learning is not necessary to provide a cause or relationship between two things but instead to be able to make a prediction. Therefore, a machine learning model might identify what constitutes a cat not by the features that we might describe to it but by a seemingly abstract set of requirements. In addition, although data sets might affect the outcome of machine learning, e.g., a data set trained only on a particular skin colour, there is no objective best way of training on any given data set. That is, the same data set might be used to create models with different levels of validity. This can be seen in machine learning competitions where standard data sets are used, such as a data set of hand written numbers, and competitors must try and create the best machine learning algorithms to interpret, with varying levels of success. The level of success may depend on various complex decision they make, such as the number of times the model is trained on that same data, the number of ‘hidden’ layers of neural networks there are, the rate of that propagation, to name but a few. The real test is whether, at the end, ‘it works’.
Machine learning may not be explainable. For example, a model that trains based on the number of times it has gone over a particular set of data cannot simply be explained by reference to a procedure and footnotes, at least in any useful fashion. To use another simple joke (which will be funny if one understands baysan inferencing): At a job interview for a machine learning expert, the Interviewer: What is 10 + 10?
Candidate : 3.
Interviewer: No, that’s wrong.
Candidate: 7.
Interviewer: No, wrong again
Candidate: 15.
Interviewer: No, that’s wrong
Candidate: 19
Interviewer: Wrong.
Candidate: 20
Interviewer: Yes, you got the job.
Just as the candidate here has a model of what 10 + 10 is, starting off hopelessly wrong (3) and gets better with time until eventually getting it right, we can assume that applying that mode to that question in the future will always result in the correct answer of 20. Where the ‘machine learning’ comes into this is that by using such a method, no-one has told the machine ahead of time what answer is. It worked it out for itself. Therefore we cannot simply open up the black box to look at the assumptions that have gone into it and work out how it makes its decision.
Instead, the way to understand how such an AI operates is to ask it a series of questions and understand what its answers are., essentially building an outside model of how the model works. For example, if there was an algorithm that was trained to identify food, you could place hotdogs before it and not hotdogs and see what it can identify. You might also then experiment by changing food from its ordinary shape and see in this instance whether it recognises a sausage sandwich as a hotdog or as a not hotdog.
What does that mean for law? The critical question now becomes: what if we are making legal decisions based on machine learning? How can we make decisions that are not explainable? First of all, we should be careful as to whether we are actually using machine learning or whether we are using a regression. Even if a statistical analysis can provide some level of accuracy in predicting the outcome of court cases or the likelihood of a person to reoffend, I have a very negative view of the utility of such system. Firstly, if you are going to make such a regression, you must prove that there is a causal relationship and that the causality runs in the correct way so that you are not saying that the appearance of umbrellas causes it to rain. A propensity for judges to find a ‘guilty verdict’ in the morning, as opposed to the afternoon, or plaintiffs with a particular name to having a better chance of success, is irrelevant. Similarly, is it that low socio-economic status causes a tendency towards criminality, or does causality run the other way?
Secondly, and more importantly, almost all of the variables that are typically used in such an analysis are irrelevant. Picking up pieces of data such as the court name, the general area of law, the solicitor firm name, the date, the jurisdiction are all highly irrelevant the actual question at hand in cases. What matters in a case is the evidence before the judge, the laws that is argued, and analysis of it in application to the facts, at the very minimum. To be blunt, any system that does not read over the words of the case and come to an understanding of them and then seek to make a decision based upon those words must surely be using irrelevant data. That is why, in my view, so-called ‘big data’ statistical analyses that proport to predict cases are a mere novelty and are little more than statistical junk. It does not matter whether these are explainable or not because they fall down for another reason altogether.